Machine learning and Data analytics are global trends. Job seekers are interested in these programs in order to advance their careers.In this blog post, we will discuss the opportunities, advantages, and duration, among other things.
Machine Learning :
Machine learning is a subset of artificial intelligence (AI) that involves the development of algorithms and models that enable computers to learn from data. Instead of being explicitly programmed to perform a task, a machine learning system uses statistical techniques to learn patterns and make predictions or decisions without explicit programming. It relies on the ability of algorithms to generalize from past experiences and improve their performance over time as they are exposed to more data. Machine learning is applied in various domains, such as image recognition, natural language processing, recommendation systems, and predictive analytics.
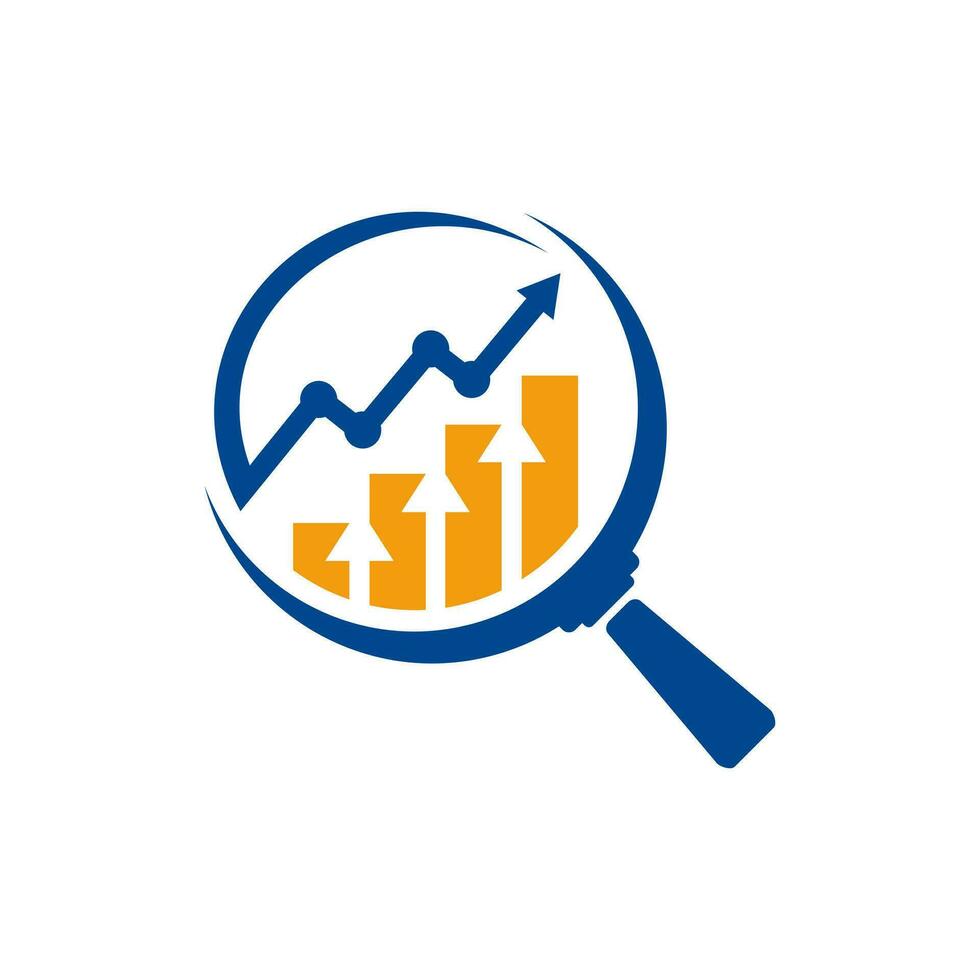
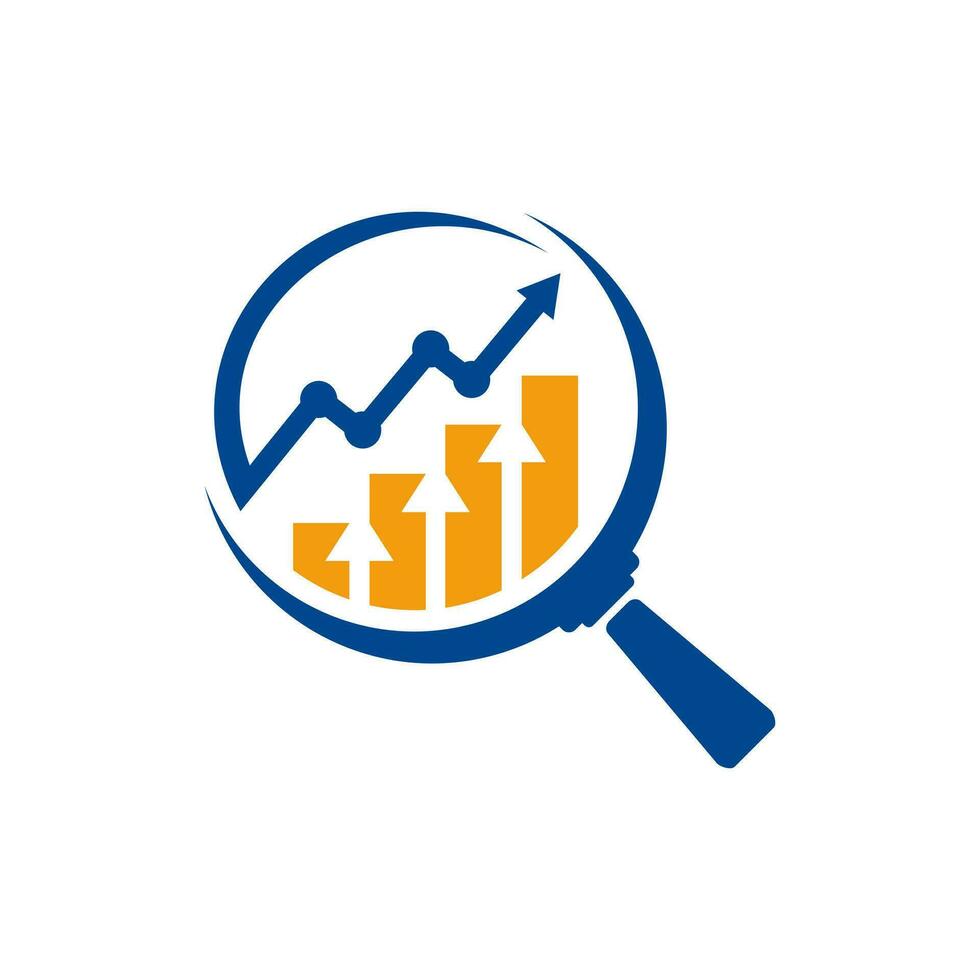
Machine Learning :
Data Analytics
Data analytics involves the analysis of data to extract meaningful insights, identify patterns, and make informed decisions. It encompasses various techniques and processes for examining and interpreting data, with the goal of uncovering valuable information that can be used for business intelligence, problem-solving, and strategic planning.
Data analytics typically involves steps such as data collection, cleaning, and transformation, followed by the application of statistical and mathematical methods to discover patterns or trends. Visualization tools are often used to present the results in a comprehensible manner. The insights gained from data analytics can be instrumental in improving business processes, optimizing strategies, and gaining a competitive advantage in various industries.
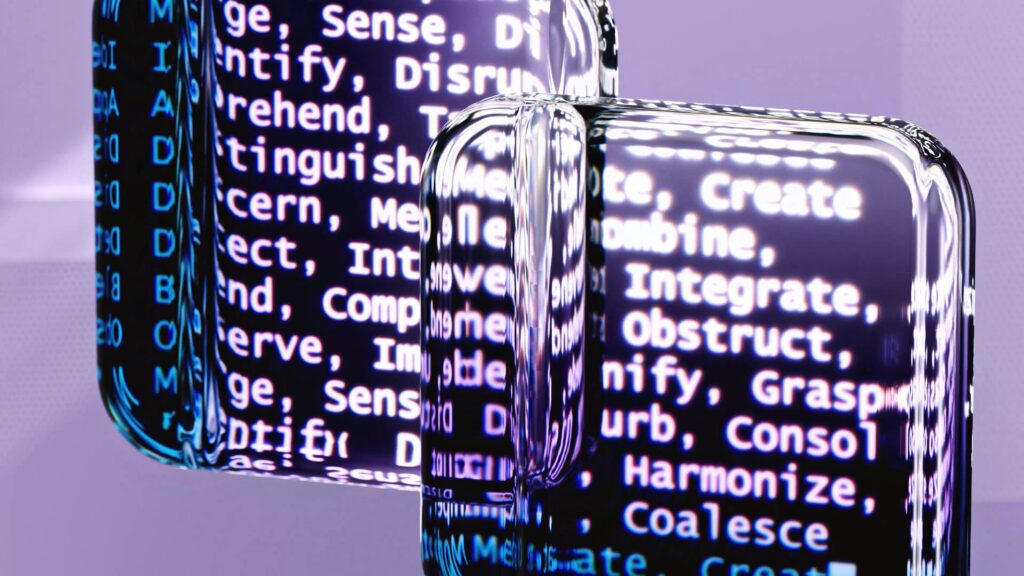
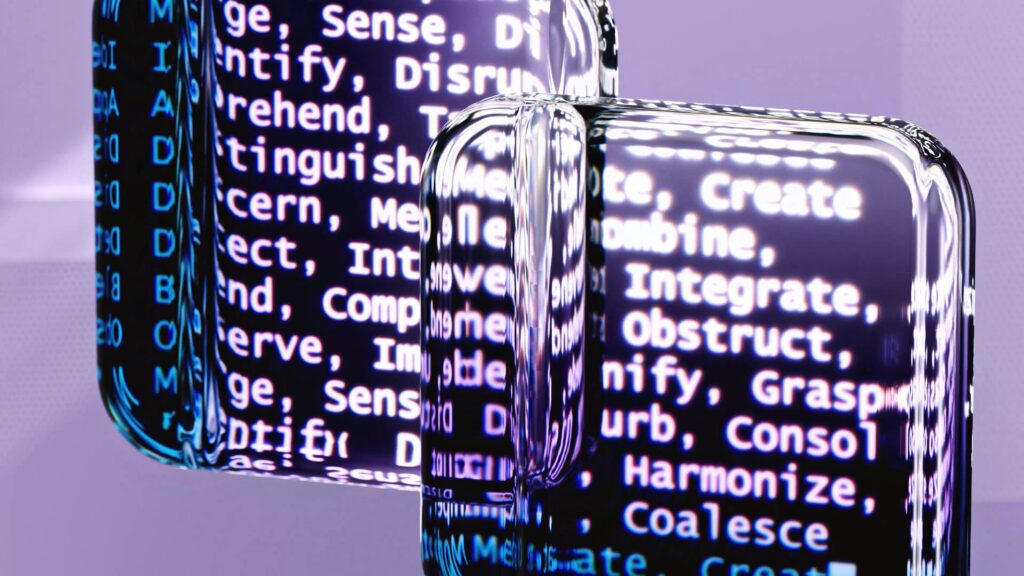
Differences:
Both machine learning (ML) and data analytics have promising futures, and their importance often depends on the specific context and industry.
Machine learning is gaining traction due to its ability to automate decision-making processes, create predictive models, and handle complex tasks such as image recognition and natural language processing. Industries like healthcare, finance, and technology are increasingly leveraging ML for innovation and efficiency.
Data analytics, on the other hand, remains crucial for extracting actionable insights from vast amounts of data. It helps organizations make data-driven decisions, optimize operations, and identify trends. As the volume of data continues to grow, the demand for skilled data analysts is likely to persist.
In essence, the synergy between machine learning and data analytics is powerful. A combination of both is often employed to maximize the value extracted from data. Learning skills in both areas can be advantageous for professionals in the evolving landscape of data-driven decision-making.
meaningful insights, identify patterns, and make informed decisions. It encompasses various techniques and processes for examining and interpreting data, with the goal of uncovering valuable information that can be used for business intelligence, problem-solving, and strategic planning.
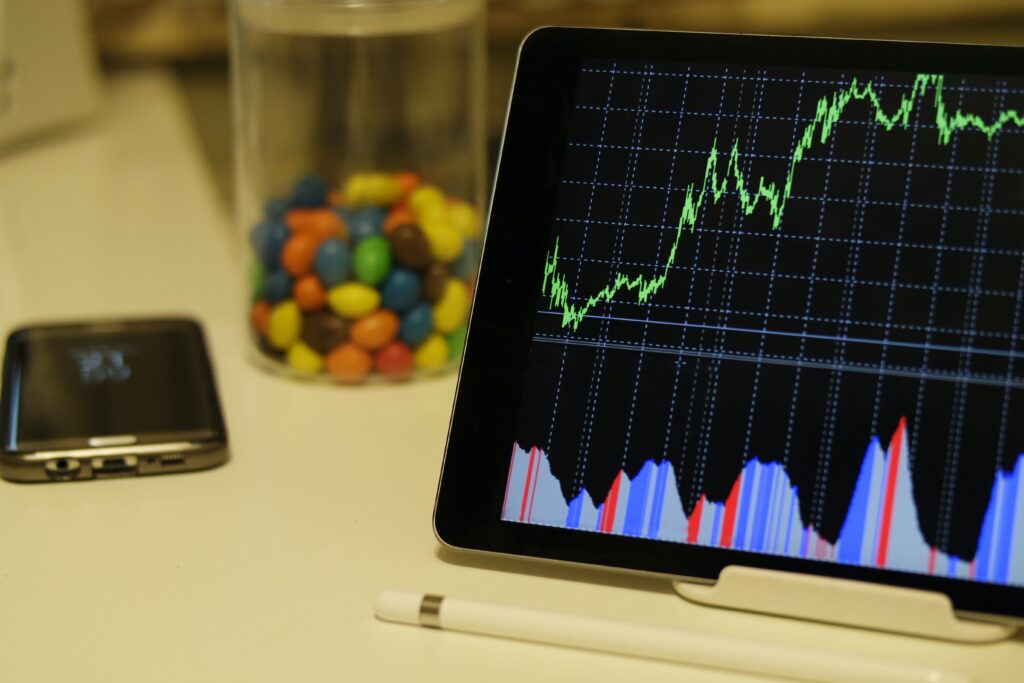
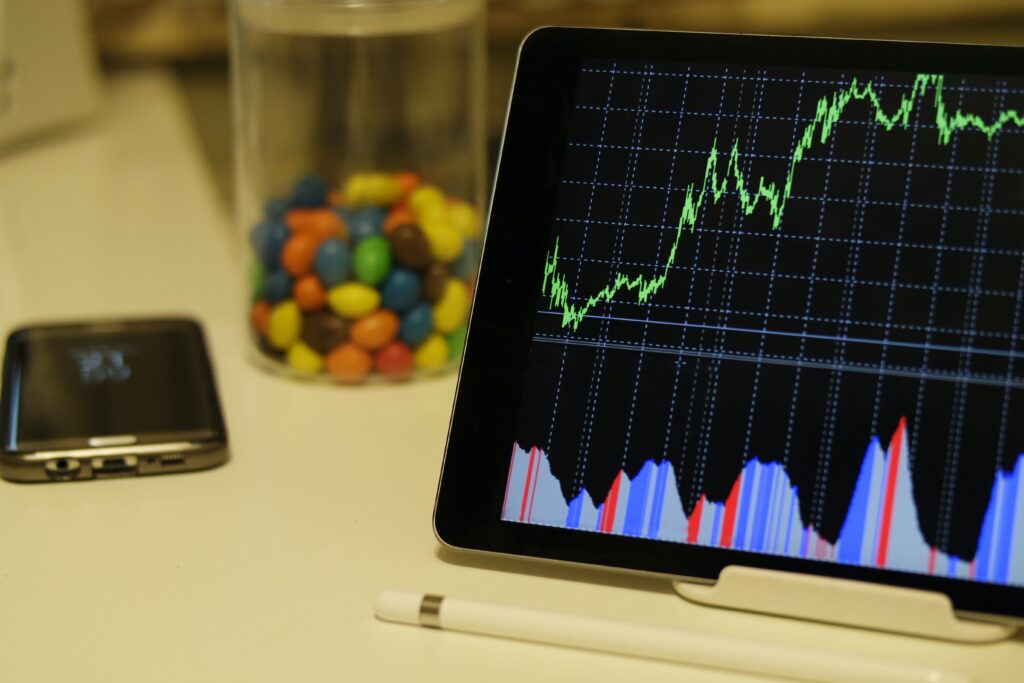
Learning duration and how difficult it is to learn
The difficulty of learning machine learning (ML) can vary depending on your background, prior experience, and the depth of understanding you aim to achieve. Here are some considerations:
- Mathematics and Programming Knowledge: ML involves concepts from linear algebra, calculus, and statistics. A solid understanding of programming (commonly using languages like Python or R) is also essential.
- Learning Resources: The availability of high-quality learning resources, tutorials, and courses has made ML more accessible. Online platforms offer a range of beginner to advanced courses.
- Practice and Projects: Practical experience is crucial. Implementing ML models and working on projects help solidify your understanding. Real-world applications often require hands-on experience.
- Complexity of Algorithms: The complexity varies. Some algorithms, like linear regression, are relatively simple to grasp, while deep learning models can be more complex. Starting with simpler algorithms and progressing gradually can make the learning curve more manageable.
- Domain-Specific Knowledge: Depending on the application domain (e.g., healthcare, finance), additional domain-specific knowledge may be necessary to apply ML effectively.
In summary, while ML can be challenging due to its mathematical underpinnings and the diversity of algorithms, many find success through gradual learning, hands-on practice, and perseverance. Starting with the basics and gradually progressing to more advanced topics can make the journey more manageable.
The difficulty of learning data analytics can vary based on several factors:
- Background Knowledge: If you have a solid foundation in statistics, mathematics, and basic programming, it can ease the learning process.
- Tools and Technologies: Data analytics often involves using tools like Excel, SQL, and data visualization tools (e.g., Tableau). Familiarity with these tools can make the learning curve less steep.
- Learning Resources: There are numerous online courses, tutorials, and resources available for learning data analytics. The quality and comprehensiveness of the learning materials can influence the ease of learning.
- Practical Experience: Hands-on experience is crucial in data analytics. Working on real-world projects, manipulating data, and deriving insights contribute significantly to skill development.
- Domain Knowledge: Depending on the industry or domain you’re interested in, having some background knowledge can be helpful. Understanding the context of the data you’re analyzing is valuable.
Overall, many find data analytics to be more accessible than some advanced areas of machine learning. It’s often considered a good starting point for individuals entering the field of data science. Practical application and continuous learning play key roles in mastering data analytics.
Duration:
The duration to learn data analytics & machine learning depends on your prior experience, learning pace, and the depth of knowledge you aim to acquire. Beginners might take a few months with consistent effort, while those with some background might learn more quickly. Continuous learning is common in this field due to evolving technologies.